Analytics-Driven Strategy Development in Professional Services
Table of Contents
- The Rise of AI and ML in Financial Services
- Enhancing Customer Experiences with AI
- AI-Driven Risk Management and Fraud Detection
- Revolutionizing Trading and Investment Strategies
- Impact on Trading
- The Impact of AI on Financial Modeling
- Regulatory Challenges and Ethical Considerations
- Future Trends: AI and the Evolving Financial Landscape
- Conclusion
In this blog, we examine the indispensable role that artificial intelligence (AI) and machine learning (ML) play in product development within the financial services industry. We begin by outlining the significant impact these technologies have on refining predictive models and decision-making processes. The discussion then shifts to how AI and ML facilitate more effective risk management strategies and fraud detection, thereby safeguarding assets and enhancing reliability. We also explore their influence on customizing client interactions and product offerings, which is vital for staying competitive in a fast-paced market. Additionally, the blog delves into the ethical and regulatory challenges that come with the implementation of these technologies, urging a balance between innovation and accountability.
Artificial Intelligence (AI) and Machine Learning (ML) continue to drive major shifts in the financial services industry, enabling innovative solutions that improve how institutions operate and interact with customers. These technologies extend beyond mere automation, touching every facet of the financial sector from personalized banking services to sophisticated risk assessment models.
The integration of AI and ML in financial services is not just about enhancing existing processes but about revolutionizing the industry. Financial institutions that adopt these technologies are shifting towards more proactive and predictive management, where decisions are informed by deep data insights and real-time analytics.
In this transformative era, AI and ML stand out as key enablers that are not just supporting but actively driving innovation in financial services, making operations more secure, efficient, and customer centric.
The Rise of AI and ML in Financial Services
The integration of AI and ML into financial services is not a recent development; it’s an evolutionary process that has matured significantly over the past decades. Initially utilized for basic data analysis and automated tasks, these technologies now underpin complex decision-making processes and innovative financial products across the sector.
Evolution
- Early Applications: One of the initial major uses of Artificial Intelligence was for credit underwriting, risk assessment, and fraud detection, specifically targeting money laundering. For example: The FinCENAuthor Artificial Intelligence system (FAIS) reviewed over 200,000 transactions per week and identified 400 potential money laundering cases worth nearly $1 billion over two years.
- Advanced Integration: AI and ML have evolved to handle sophisticated functions such as real-time fraud detection, dynamic risk assessment, and automated trading systems.
Growth and Adoption
- Adoption Rates: The adoption of AI in financial services has seen exponential growth. As of the latest surveys, over 75% of banks with assets greater than $100 billion are deploying AI technologies for various operational enhancements.
- Impact Statistics: Financial institutions that integrate AI report up to a 30% increase in efficiency in processes like customer service and transaction monitoring.
Transform your financial services with AI and ML
Enhancing Customer Experiences with AI
In the realm of financial services, AI has become a pivotal force in transforming customer interactions and service delivery. By adapting machine learning algorithms, banks and financial institutions can offer highly personalized services that were unimaginable a decade ago.
Personalization in Customer Service
- Chatbots and Virtual Assistants: AI-powered chatbots are now standard in online banking environments, providing 24/7 customer service that can handle inquiries, troubleshoot issues, and guide users through complex financial processes without human intervention.
- Personalized Financial Advice: Advanced ML models analyze individual customer data to provide customized financial advice. For example, suggesting budget plans or investment options based on personal spending habits and financial goals.
Examples of AI in Action
- Chatbots Enhancing Accessibility: Major banks have deployed AI-driven chatbots that can perform tasks ranging from checking account balances to initiating wire transfers, significantly reducing wait times and freeing human agents to handle more complex queries.
- Tailored Product Offerings: By analyzing customer data, financial institutions can offer personalized loan rates and credit card offers, which not only meet the specific needs of customers but also improve conversion rates.
AI-Driven Risk Management and Fraud Detection
The deployment of AI and ML in risk management and fraud detection represents a quantum leap in how financial institutions safeguard their operations and their clients’ assets. By employing sophisticated algorithms, these technologies are not just reactive but also proactive in identifying and mitigating potential threats.
Enhancement of Risk Prediction and Management
- Predictive Risk Modeling: AI systems analyze historical data and predict future outcomes, allowing banks to adjust their risk models in real time and respond more effectively to emerging threats.
- Credit Risk Assessment: ML models are increasingly used to refine the accuracy of credit scoring by incorporating a broader range of data points, including non-traditional variables that improve prediction of borrowers’ behavior.
Fraud Detection through AI
- Real-Time Detection: AI algorithms analyze transaction patterns on a scale and speed unattainable for human monitors, spotting irregularities that may indicate fraudulent activities almost instantly.
- Behavioral Biometrics: Institutions are now using ML to analyze not just financial transactions but also the way a user interacts with banking interfaces, which can help detect fraud through unusual behavior patterns.
Real-World Application
TIBCO’s Fraud Detection on Azure
A standout example of AI-driven fraud detection in action involves TIBCO’s use of Microsoft Azure. TIBCO has developed the Connected Intelligence Platform that utilizes advanced analytics and AI to enhance fraud detection capabilities in financial services. This platform, built on Azure, helps financial institutions manage the rising challenges of fraud which have become more complex with the diversification and speed of modern financial transactions.
The Connected Intelligence Platform employs AI to augment human decision-making, allowing analysts to focus on the most suspicious transactions by processing and analyzing vast amounts of data in real time. This integration of AI significantly lowers costs, improves risk management, and enhances customer satisfaction by increasing the accuracy of fraud predictions. The system uses cloud-native technologies like Azure Event Hub and Azure IoT Hub for real-time streaming analytics and event processing, and applies both supervised and unsupervised machine learning models to generate fraud probability scores (Microsoft Cloud).
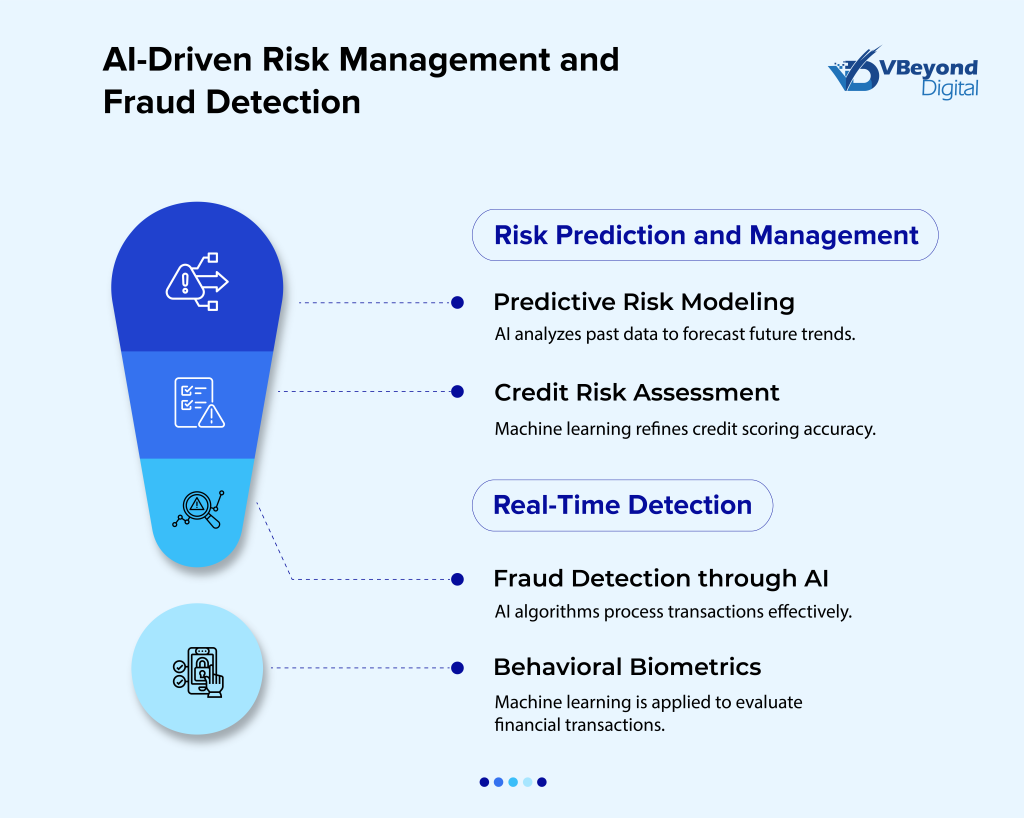
Revolutionizing Trading and Investment Strategies
AI has dramatically transformed trading and investment strategies, enabling financial institutions to execute high-frequency trading and manage portfolios with unprecedented precision and speed.
Algorithmic Trading
- Automated Trading Systems: AI and ML are central to the development of algorithmic trading, where computers execute trades at speeds and volumes that surpass human capabilities. These systems analyze market data to make instantaneous trading decisions based on predefined criteria.
- Enhanced Market Analysis: AI tools process and interpret vast arrays of historical and real-time market data to identify trends that might be invisible to human analysts, allowing for more informed and timely trading decisions.
Real-Time Data Processing
- Portfolio Management: AI-driven tools help portfolio managers assess risk, diversify investments, and optimize asset allocation by analyzing market conditions and individual asset performance in real time.
- Market Trend Prediction: Advanced predictive analytics powered by ML can forecast market movements based on complex scenarios, incorporating economic indicators, company performance metrics, and consumer behavior patterns.
Impact on Trading
The impact of AI on trading can be profound, as evidenced by:
- Efficiency and Speed: AI systems can analyze multiple markets simultaneously, reacting to changes instantaneously and executing trades within milliseconds.
- Risk Reduction: AI’s predictive capabilities enhance risk assessment, allowing traders to mitigate potential losses more effectively.
Real-World Applications
- MyGKone and Azure: MyGKone utilized Azure AI to develop a robust, real-time monitoring system that significantly enhances transaction analysis, allowing for quick identification of market opportunities and risks. This system is pivotal in maintaining market relevance and competitive edge in the volatile financial market landscape. For more information, view the MyGKone case study.
- Robeco and Azure Monitor: Robeco leveraged Azure Monitor to enhance its investment strategies by providing real-time analytics and deeper insights into operational data, which enables better decision-making and a more strategic allocation of resources in portfolio management. Detailed information on their implementation can be found in the Robeco case study.
The Impact of AI on Financial Modeling
AI is revolutionizing the way financial models are constructed, enabling institutions to simulate and predict a wide array of economic scenarios with remarkable accuracy and detail.
Role of AI in Financial Modeling
- Evaluating Models: AI can evaluate financial models that incorporate a multitude of variables, including economic indicators, market trends, and consumer behavior. These models can generate detailed forecasts and scenarios to support strategic planning and decision-making.
- Enhancing Predictive Capabilities: Through deep learning and neural networks, AI improves the predictiveness of financial models, allowing financial analysts to anticipate market movements and potential disruptions more effectively.
Preparing for Market Changes
- Scenario Analysis and Stress Testing: Financial institutions use AI to perform stress tests and scenario analyses, assessing the impact of various hypothetical events on their portfolios and operations. This helps in preparing robust response strategies.
- Dynamic Adaptation to Market Conditions: AI enables models to adapt dynamically to changing market conditions, providing financial institutions with the agility to respond to unforeseen events swiftly and effectively.
Real-World Impact
- London Stock Exchange Group (LSEG) and Microsoft: LSEG and Microsoft have formed a strategic partnership to co-innovate solutions for the financial markets, integrating LSEG’s comprehensive data and analytics with Microsoft’s global cloud platform and AI capabilities. This collaboration is transforming financial services workflows, improving decision-making, communications, and productivity while maintaining regulatory compliance. Their combined efforts aim to deliver more connected solutions, offering greater efficiency, resilience, and scalability across all workflows.
For more detailed insights, you can read the full discussion on the partnership between LSEG and Microsoft here.
Regulatory Challenges and Ethical Considerations
The widespread adoption of AI in financial services is not without its challenges, particularly in the realms of regulatory compliance and ethical considerations. As financial institutions integrate more AI-driven processes, they must navigate a complex landscape of laws and ethical dilemmas to ensure responsible and fair use of these technologies.
Regulatory Landscape
- Compliance with Global Standards: Financial institutions must adhere to a variety of international regulations designed to ensure the security and integrity of financial systems, such as GDPR (General Data Protection Regulation) in Europe and Dodd-Frank in the United States. AI implementations must be transparent and compliant with these and other regulatory frameworks.
- Regular Audits and Reporting: AI systems require regular audits to ensure they perform as intended without introducing biases or errors. These audits help maintain compliance and build trust among stakeholders and customers.
Ethical Implications
- Bias and Fairness: AI systems are only as unbiased as the data they are trained on. Ensuring that AI models do not perpetuate existing biases or create new ones is crucial for maintaining fairness in financial decision-making.
- Transparency and Explainability: There is a growing demand for AI systems to be explainable, meaning that their decisions can be understood and justified to customers and regulators. This is particularly important in financial services, where decisions can significantly impact customers’ lives and financial well-being.
Navigating Challenges
- Implementing Ethical AI Frameworks: Many institutions are now developing ethical AI frameworks to guide the development and deployment of AI technologies. These frameworks often include principles like fairness, accountability, and transparency.
- Collaboration with Regulators: Proactive collaboration with regulatory bodies can help ensure that AI systems are not only compliant but also aligned with broader societal values and customer expectations.
Future Trends: AI and the Evolving Financial Landscape
As we look to the future, AI is set to continue its transformative impact on the financial sector, not only through advancements in existing technologies but also through the emergence of new applications and integration with other cutting-edge technologies.
Prediction of Future AI Trends
- Development of Super Apps: Financial services are likely to see the rise of ‘super apps’ that integrate banking, investment, insurance, and other financial services into a single platform, driven by AI to provide seamless, personalized user experiences.
- Integration with Blockchain and IoT: AI’s convergence with blockchain technology and the Internet of Things (IoT) promises enhanced security, improved transparency, and better customer engagement. These integrations are expected to further revolutionize payments, asset management, and compliance.
Long-Term Implications
- Automated and Real-Time Decision Making: With continuous improvements in AI and incremental learning capabilities, future financial systems are likely to operate with minimal human intervention, making real-time decisions that optimize financial outcomes and customer satisfaction.
- Global Financial Systems Transformation: AI is poised to redefine global financial systems, promoting greater financial inclusion and democratizing access to financial services across different geographies and demographics.
Conclusion
Strategically incorporating and expanding the use cases of AI and ML in financial services can offer big payoffs. As we have explored, AI and ML are not merely technological enhancements but are pivotal in driving significant advancements in customer service, risk management, trading strategies, and financial modeling.
Key Takeaways:
- Transformative Potential: AI and ML have reshaped every facet of financial services, from customer interactions to complex financial decision-making processes.
- Need for Innovation: Continuous innovation in AI technologies is crucial for maintaining competitiveness and addressing the evolving needs of consumers and markets.
- Ethical and Regulatory Considerations: As financial institutions look to harness the power of AI, they must also navigate ethical and legal concerns and regulatory challenges to ensure that these technologies benefit all stakeholders without compromising fairness or privacy.
The integration of AI in financial services promises not only enhanced efficiency and security but also a more inclusive financial ecosystem that can adapt to the needs of a global customer base. By embracing these technologies, financial institutions can look forward to not only surviving but thriving in the dynamic landscape of global finance.
As we continue to innovate and adapt, the role of AI and ML in financial services will undoubtedly expand, bringing about further changes that we can only begin to anticipate. The future of finance is bright, and it is digital, driven by the intelligent algorithms that we have only started to deploy.