Predictive Analytics in Financial Services with Power BI: A Path to Personalization and Efficiency
Table of Contents
- Understanding Power BI and Its Capabilities in Predictive Analytics
- Business Efficiency with Power BI’s Predictive Capabilities: Enterprise Deployment Examples
- Challenges in Implementing Predictive Analytics with Power BI
- Power BI’s Role in Risk Management and Compliance
- Best Practices for Implementing Power BI Predictive Analytics
- Conclusion
In financial services, the application of predictive analytics in finance is revolutionizing how institutions operate and serve their clients. This detailed exploration dives into the powerful capabilities of Power BI to facilitate these advancements. Readers will gain insights into how predictive analytics can refine risk assessment processes, tailor financial products to individual customer needs, and streamline operations. The guide covers practical implementation strategies, showcases examples from industry leaders, and provides actionable advice to help financial professionals make informed decisions. By integrating Power BI, financial organizations can not only improve their analytical processes but also enhance client satisfaction and operational productivity, paving the way for a more adaptive and intelligent financial environment.
The integration of predictive analytics in financial services signifies a pivotal shift towards more data-driven operations and strategy decisions. The role of advanced analytical tools such as Power BI is pivotal in this evolution, offering extensive capabilities that extend well beyond basic data analysis to support sophisticated financial decision-making processes.
Power BI differentiates itself with its comprehensive feature set, specifically designed to meet the complex needs of financial data analytics. Its ability to seamlessly integrate vast datasets and execute real-time analytics equips financial institutions with the necessary tools for informed, predictive decision-making. This blog explores the specific benefits of Power BI’s capabilities to bring more personalization to delivery of services to customers and optimize costs.
Understanding Power BI and Its Capabilities in Predictive Analytics
Power BI is distinguished by its robust feature set and integration options, designed to accommodate the multifaceted demands of financial data analytics tools. Its prowess in integrating vast datasets, coupled with the ability to perform real-time analytics, empowers financial institutions with AI-enhanced insights that are both actionable and predictive. Unlike other analytics tools, Power BI excels in processing and visualizing financial data, offering a versatile platform that simplifies complex analytics tasks.
Key features include:
- Data Integration: Seamless assimilation of data from diverse sources, ensuring a unified analytics experience.
- Real-Time Analytics: Capability to process and analyze data as it is received, ideal for dynamic financial environments.
- AI-Enhanced Insights: Advanced algorithms that provide predictive outputs, helping foresee market trends and customer behavior.
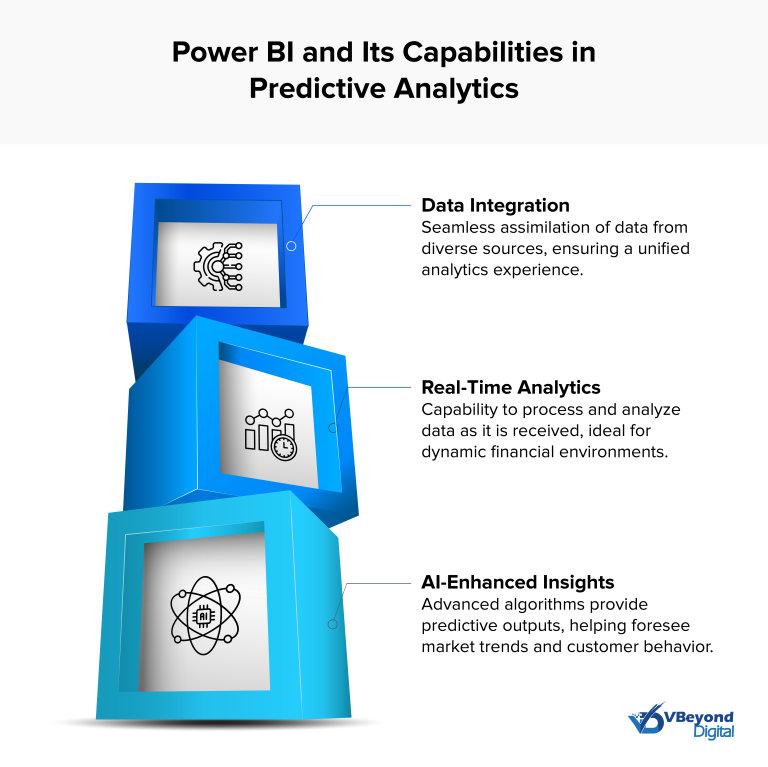
Business Efficiency with Power BI’s Predictive Capabilities: Enterprise Deployment Examples
Power BI’s predictive analytics features and detailed report formats in finance dramatically transforms the personalization in financial services. By harnessing data-driven insights, financial institutions can create highly customized service offerings that resonate with individual customer preferences and financial behaviors.
Examples of Enterprises Improving Service Delivery
- Metro Bank’s Customer Insight: Metro Bank leverages Power BI to consolidate customer transaction data across various platforms—mobile, online, and in-person—allowing for a unified view of customer activities. This comprehensive data integration enables Metro Bank to optimize customer interactions and personalize banking experiences based on individual behaviors and preferences. The use of Power BI has transformed their customer service by enabling the creation of over 100 reports that track everything from account activities to customer feedback, thereby enhancing decision-making processes and customer satisfaction. Read more about this case study here.
- Microsoft Treasury’s Risk Management: In another example, Microsoft’s Treasury uses Power BI to monitor and analyze financial data across its global operations. This allows them to respond proactively to changes in cash flow and investment returns. The predictive analytics capabilities of Power BI enable the team to foresee financial risks and adjust strategies, accordingly, ensuring robust risk management and personalized financial oversight. Explore this case study in detail here.
Examples of Improved Efficiency Achieved
- Fortune 500 Financial Institution: This institution faced challenges with manual, time-intensive reporting processes. By partnering with phData and implementing Power BI along with Snowflake, they transformed their reporting workflows into automated, on-demand processes. This shift significantly reduced report development time by 35%, decreased report failure rates by 25%, and allowed for the creation of three new reports during the engagement period. The full implementation also involved extensive training for developers and financial analysts, fostering a data-centric culture within the organization. Learn more about this case study.
- Major Facilities Management Corporation: Apex Systems helped this corporation transition from manual data processing to automated daily reporting using Power BI. This change was implemented within seven weeks and included the development of 23 report pages within a Power BI application, which replaced the previous cumbersome and time-consuming manual processes. The automation provided by Power BI enabled nightly data refreshes and a significant improvement in the usability and functionality of the reporting process, highlighting key performance indicators across various departments. Read more about this transformation.
Learn how Power BI can transform your predictive analytics.
Challenges in Implementing Predictive Analytics with Power BI
While the integration of Power BI into financial services offers significant advantages, it also presents certain predictive analytics challenges that organizations must navigate carefully. Addressing these issues is crucial for maximizing the benefits of predictive analytics.
Common Challenges in Implementation
- Data Complexity: Financial data is often complex and voluminous, posing significant challenges in data management and analysis. Power BI helps manage this complexity, but the initial setup and ongoing data governance require careful planning and expertise.
- Scalability Issues: As financial institutions grow, their data analytics tools must scale accordingly. Power BI offers scalability, yet transitioning existing systems to this scalable solution can be a daunting task.
- User Adoption: Despite its powerful capabilities, the adoption of Power BI can be hindered by its learning curve. Ensuring that staff are adequately trained and understand how to use Power BI effectively is essential for realizing its full potential.
Strategies for Overcoming These Challenges
- Invest in Training: Providing comprehensive training and resources to help employees understand and utilize Power BI will facilitate smoother adoption.
- Implement Data Governance: Establishing strong data governance practices will help manage the complexity and volume of financial data.
- Plan for Scalability: Designing systems with scalability in mind from the outset will help mitigate potential future disruptions as demand increases.
Power BI’s Role in Risk Management and Compliance
Predictive analytics in financial services, particularly through power bi predictive models, play a crucial role in risk management and compliance. This technology aids financial institutions in identifying potential risks and compliance issues before they escalate, thereby safeguarding against financial losses and regulatory penalties.
How Power BI Enhances Risk Management and Compliance
- Risk Assessment: Power BI’s predictive models analyze trends and patterns from historical data to forecast potential risks. This approach allows firms to adjust their strategies accordingly, reducing the likelihood of adverse outcomes.
- Fraud Detection: By implementing anomaly detection algorithms, Power BI helps identify unusual transactions that may indicate fraudulent activity, enabling quicker responses to such threats.
- Regulatory Compliance: Power BI can be programmed to monitor compliance with various regulatory requirements. It automatically updates these checks based on new regulations, ensuring that financial institutions remain compliant.
Real-Life Impact
Power BI’s capabilities in real-time fraud detection are significantly demonstrated through its integration with Azure services. For instance, Azure’s architecture for real-time fraud detection involves components like Azure Blob Storage, Azure Event Hubs, and Azure Stream Analytics. This setup allows organizations to process data in real-time to identify fraudulent transactions efficiently. The scenario uses a predefined query in Azure Stream Analytics to analyze data streams for anomalous activities, which helps in the rapid detection and response to potential fraud (Microsoft Learn).
Additionally, TIBCO has used Microsoft Azure to achieve breakthroughs in fraud detection. Their Connected Intelligence platform combines AI with real-time streaming analytics, providing financial institutions with tools to enhance fraud detection. This platform processes massive volumes of data to identify potentially fraudulent transactions quickly, helping to reduce costs and improve customer satisfaction. The AI-driven approach augments human decision-making, focusing analysts’ efforts on transactions most likely to be fraudulent, thereby improving the accuracy and efficiency of fraud detection processes (Microsoft Cloud).
For insights on TIBCO’s use of Azure for intelligent fraud detection, you can read further here: TIBCO creates a fraud detection breakthrough on Azure.
Best Practices for Implementing Power BI Predictive Analytics
Implementing Power BI for predictive analytics in financial services involves a systematic approach to integrate advanced data analytics into the core operational framework. By following these guidelines, firms can ensure they maximize the utility of Power BI’s capabilities while aligning with broader business objectives.
Step-by-Step Guide for Adopting Power BI
- Assessment and Planning:
Identify Business Needs: Determine the specific business processes and decisions that could benefit from predictive analytics.
Infrastructure Evaluation: Assess the existing IT infrastructure and data governance practices to ensure compatibility with Power BI requirements. - Data Integration and Management:
Data Collection: Aggregate data from various sources, including internal systems and external feeds.
Data Preparation: Cleanse and organize data to ensure accuracy and reliability for analytics. - Implementation and Deployment:
Pilot Testing: Start with a pilot project that addresses a significant but manageable business area to gauge the impact of predictive analytics.
Full-scale Deployment: Roll out the analytics solution across all relevant areas of the business following successful testing and feedback incorporation. - Advanced Analytics Deployment:
Model Development:
Develop predictive models using Power BI’s machine learning capabilities.
Continuous Improvement: Regularly update models based on new data and feedback to refine predictions and outcomes.
Aligning Power BI Deployment with Business Objectives
- Strategic Integration: Ensure that the implementation of Power BI predictive analytics aligns with the strategic goals of the organization, such as increasing profitability, enhancing customer satisfaction, or reducing risk.
- Stakeholder Engagement: Involve key stakeholders from various departments early in the process to align the analytics objectives with departmental and overall business goals.
- Performance Metrics: Establish clear metrics to measure the success of the Power BI initiative in terms of return on investment, customer impact, and operational efficiency.
Conclusion
Power BI’s predictive analytics capabilities bring transformative potential to the financial services sector, enhancing personalization, increasing operational efficiency, overcoming implementation challenges, and fortifying risk management and compliance practices. By adopting Power BI, financial institutions can better understand their customers’ needs and deliver customized services and solutions.